Easy interface for obtaining fits of 2D data.
The purpose of this package is to provide a very simple interface to obtain some of the most common fits of 2D data. Currently, simple fitting functions are available for linear, quadratic, cubic, exponential, and spline fits.
On the background this interface uses the LsqFit and Interpolations, which are already quite easy to use. Additionally, EasyFit contains a simple globalization heuristic, such that good non-linear fits are obtained often.
Our aim is to provide a package for quick fits without having to think about the code.
julia> ] add EasyFit
julia> using EasyFit
Read the Linear fit
section first, because all the others are similar, with
few specificities:
- Linear fit
- Quadratic fit
- Cubic fit
- N-th degree fit
- Exponential fits
- Splines
- Moving Averages
- Density function
- Bounds
- Example output:
- Options
To perform a linear fitting, do:
julia> x = sort(rand(10)); y = sort(rand(10)); # some data
julia> fit = fitlinear(x,y)
------------------- Linear Fit -------------
Equation: y = ax + b
With: a = 0.9245529646308137
b = 0.08608398402393584
Pearson correlation coefficient, R = 0.765338307304594
Predicted y = [-0.009488291459872424, -0.004421217036880542...
Residues = [-0.08666886144188027, -0.12771486789744962...
--------------------------------------------
The fit
data structure which comes out of fitlinear
contains the output data with
the same names as shown in the above output:
julia> fit.a
0.9245529646308137
julia> fit.b
0.08608398402393584
julia> fit.R
0.765338307304594
The fit.x
and fit.y
vectors can be used for plotting the results:
julia> using Plots
julia> scatter(x,y) # the original data
julia> plot!(fit.x,fit.y) # the fit
Use the fitquad
function:
julia> fitquad(x,y) # or fitquadratic(x,y)
------------------- Quadratic Fit -------------
Equation: y = ax^2 + bx + c
With: a = 0.935408728589832
b = 0.07985866671623199
c = 0.08681962205579699
Pearson correlation coefficient, R = 0.765338307304594
Predicted y = [0.08910633345247763, 0.08943732276526263...
Residues = [0.07660191693062993, 0.07143385689027287...
-----------------------------------------------
Use the fitcubic
function:
julia> fitcubic(x,y)
------------------- Cubic Fit -----------------
Equation: y = ax^3 + bx^2 + cx + d
With: a = 1.6860182468269271
b = -2.197790204605215
c = 1.431666717127516
d = -0.10389199522825227
Pearson correlation coefficient, R = 0.765338307304594
Predicted Y: ypred = [0.024757602237563042, 0.1762724543346461...
residues = [-0.021614675301217884, 0.0668157091306878...
-----------------------------------------------
Use the fitndgr
function:
julia> fitndgr(x,y,4)
------------- n-th degree polynomial degree Fit -------------
Equation: y = sum(p[i] * x^(i-1) for i in n+1:-1:1)
With: p = [1.0000000000011207, 1.99999999996782, 3.0000000001850315, 3.999999999655522, 6.000000000197637]
Pearson correlation coefficient, R = 1.0
Average square residue = 2.2956403558488966e-25
Predicted Y: ypred = [ 1.036097252072566, 1.23390364829286, ...]
residues = [ 6.104006189389111e-13, -5.706546346573305e-13, ...]
-------------------------------------------------------------
Use the fitexp
function:
julia> fitexp(x,y) # or fitexponential
------------ Single Exponential fit -----------
Equation: y = A exp(x/b) + C
With: A = 0.08309782657193134
b = 0.4408664103095801
C = 1.4408664103095801
Pearson correlation coefficient, R = 0.765338307304594
Predicted Y: ypred = [0.10558554154948542, 0.16605481935145136...
residues = [0.059213264010704494, 0.056598074147493044...
-----------------------------------------------
or add n=N
for a multiple-exponential fit:
julia> fit = fitexp(x,y,n=3)
-------- Multiple-exponential fit -------------
Equation: y = sum(A[i] exp(x/b[i]) for i in 1:3) + C
With: A = [2.0447736471832363e-16, 3.165225832379937, -3.2171314371600785]
b = [0.02763465220057311, -46969.25088088338, -4.403370258345724]
C = 3.543252432454542
Pearson correlation coefficient, R = 0.765338307304594
Predicted Y: ypred = [0.024313571992034433, 0.1635108558614995...
residues = [-0.022058705546746493, 0.05405411065754118...
-----------------------------------------------
The fitting of splines requires the use of the Interpolations
package
(this explicit requirement was introduced in version 0.6 of EasyFit
,
and depends on julia >= 1.9
).
To use the fitspline
function, do:
julia> using EasyFit, Interpolations
julia> fit = fitspline(x,y)
-------- Spline fit ---------------------------
x spline: x = [0.10558878272489601, 0.1305310750202113...
y spline: y = [0.046372277538780926, 0.05201906296544364...
-----------------------------------------------
Use plot(fit.x,fit.y)
to plot the spline.
Use the movavg
(or movingaverage
) function:
julia> ma = movavg(x,50)
------------------- Moving Average ----------
Number of points averaged: 51 (± 25 points)
Pearson correlation coefficient, R = 0.9916417123050962
Averaged Y: y = [0.14243985510210114, 0.14809841636897675...
residues = [-0.14230394758154755, -0.12866864179092025...
--------------------------------------------
Use plot(ma.x,ma.y)
to plot the moving average.
Use the fitdensity
to obtain the density function (continuous histogram)
of a data set x
:
julia> x = randn(1000)
julia> density = fitdensity(x)
------------------- Density -------------
d contains the probability of finding data points within x ± 0.25
-----------------------------------------
Options are the step size (step=0.5
) and normalization type
(probability by default, with norm=1
or number of data points,
with norm=0
).
Example:
julia> x = randn(1000);
julia> density = fitdensity(x,step=0.5,norm=0);
julia> histogram(x,xlabel="x",ylabel="Density",label="",alpha=0.3,framestyle=:box);
julia> plot!(density.x,density.d,linewidth=2);
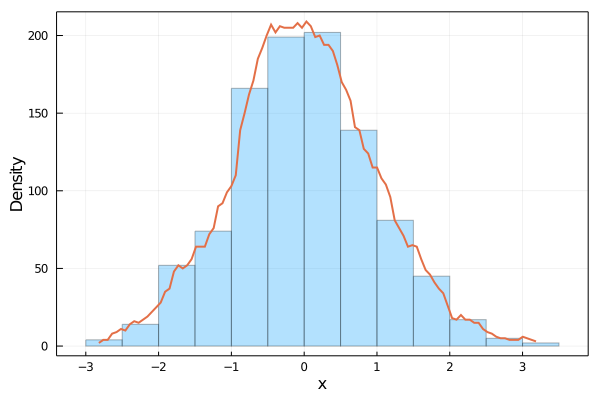
Lower and upper bounds can be set to the parameters of each function using the
l=lower()
and u=upper()
input parameters. For example:
julia> fit = fitlinear(x,y,l=lower(a=5.),u=upper(a=10.))
julia> fit = fitexp(x,y,n=2,l=lower(a=[0.,0]),u=upper(a=[1.,1.]))
Bounds to the intercepts or limiting values are not supported, but it is possible to set them to a constant value. For example:
julia> fit = fitlinear(x,y,b=5.)
julia> fit = fitexp(x,y,n=2,c=0.)
This figure was obtained using Plots
, after obtaining a fit of each type, with
julia> scatter!(x,y) # plot original data
julia> plot!(fit.x,fit.y) # plot the resulting fit

The complete script is available at: plots.jl
It is possible to pass an optional set of parameters to the functions. Use, for example:
julia> fitexp(x,y,options=Options(maxtrials=1000))
Available options:
Keyword | Type | Default value | Meaning |
---|---|---|---|
fine |
Int |
100 | Number of points of fit to smooth plot. |
p0_range |
Vector{Float64,2} |
[-100*(maximum(Y)-minimum(Y)), 100*(maximum(Y)-minimum(Y))] |
Range of generation of initial random parameters. |
nbest |
Int |
5 | Number of repetitions of best solution in global search. |
besttol |
Float64 |
1e-4 | Similarity of the sum of residues of two solutions such that they are considered the same. |
maxtrials |
Int |
100 | Maximum number of trials in global search. |
debug |
Bool |
false | Prints errors of failed fits. |